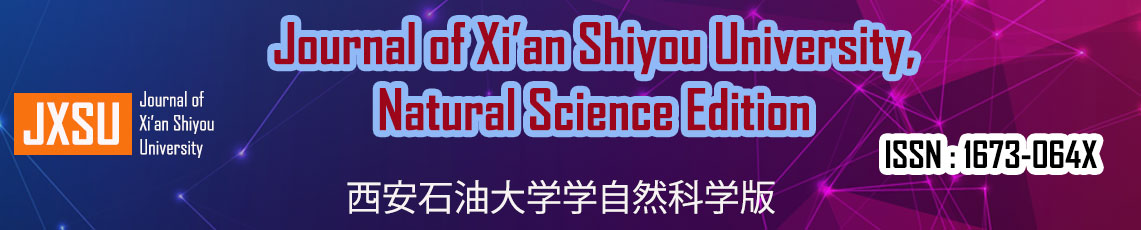
Home / Articles
Prediction Of Chronic Kidney Disease Using Different Classification Algorithms: A Comparative Study.
Kidney disease (KD) is a serious public health concern is defined as a disorder that disrupts the kidneys' normal function. As the rate of people influenced by CKD continues to rise, efficient prediction techniques should be considered. Therefore, the goal of this work is to help in the prevention of Chronic Kidney Disease (CKD) applying machine learning approaches to detect CKD early on and keep chronic kidney disease from getting worse. In this paper, we focus on applying various classification algorithms perform on a dataset of 400 patients with 24 variables linked to chronic kidney disease diagnosis in aims to compare the performance of these algorithms in making predictions and justify the algorithm that achieves more correct prediction. The classification techniques used in this study include Random Forest (RF), Decision Tree (DT), and Naive Bayes (NB). To perform experiments, all missing and outlier values in the dataset were replaced by the mean of the corresponding attributes. Next, in the feature selection step, we optimizing the prediction model and obtaining the optimal subset of features from the dataset. In the proposed approach, correlation coefficient, and recursive feature elimination have been used as feature selection methods to decrease the number of features by eliminating irrelevant and useless features to maintain a good analytical result. Finally, different assessment parameters are used to evaluate the classifiers' performance such as Accuracy, Precision, Recall, and F1-score. The empirical results from the experiments serve that RF performed better than DT, and NB with accuracies of 100% in CKD predicting.
Keywords - Kidney disease; prediction systems; Random Forest; Decision Tree; Naive Bayes.