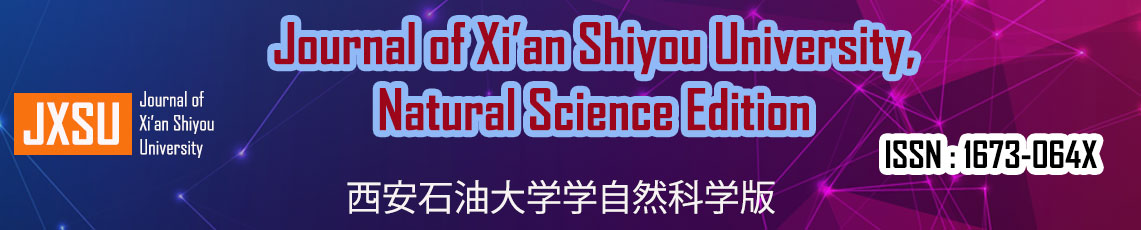
Home / Articles
AN EFFICIENT APPROACH TO PREDICT CORONARY HEART DISEASE USING DEEP NEURAL NETWORK
Every year, the population of people with coronary heart disease (CHD) grows, and a considerable number of people die from it. Furthermore, The misdiagnosis of coronary heart disease is a critical fundamental that caused death. Thus, to avoid misdiagnosis of CHD, artificial methods are designed to assist medical practitioners in making the proper decision and diagnosing CHD in its early stages. This paper proposed a new efficient model based on Deep Neural Network (DNN) which can be used to diagnose coronary heart disease in better performance than the traditional diagnostic methods. This research developed a new efficient model based on Deep Neural Network (DNN) that can be utilized to identify coronary heart disease more effectively than previous traditional diagnostic methods. Some researchers have created a system that uses a traditional neural network or another machine learning technique, but the results were not effective. In this paper, The proposed model is constructed using a Deep Neural Network (DNN), which is based on a standard neural network with a different number of hidden layers. The neural network is a supervised learning algorithm that excels in classification tasks. The binary classification was used in the DNN model to diagnose if CHD was present (representative "1") or missing (representative "0"). To aid performance analysis, we used the Cleveland heart disease dataset from the UCI machine learning repository that consists of 13 features and 303 cases. Also, a comparative study of the performance of various machine learning algorithms like KNN, SVM, Naïve Bayes is done in an aim to demonstrate the effectiveness of the DNN algorithm in CHD prediction. The experimental results reveal that out of these four classification models, the DNN method can predict CHD with the highest accuracy of 96% when using four layers and 350 epoch.
Keywords: Coronary Heart Disease, Deep Neural Network, Diagnostic models, UCI Machine Learning Repository.