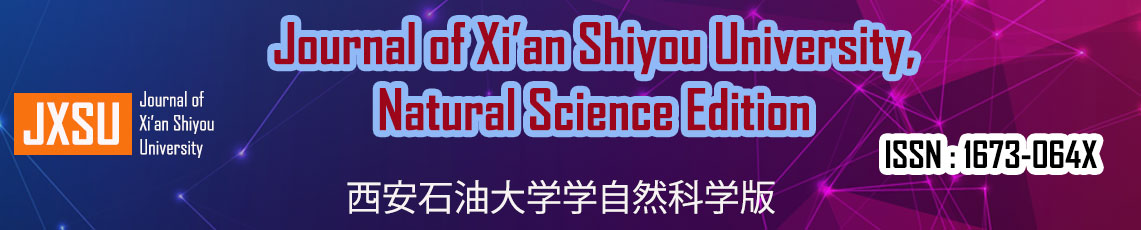
Home / Articles
Malware Classification Using Customized Convolutional Neural Networks by Leaky RELU Activated Function
In most recent years, the quantity of malware has increased enormously, raising a significant security risk to financial organizations, businesses and individuals. In order to analyze and identify such malware effectively, we need to be able to generalize them into groups and define their corresponding families based on their behavior. In this paper, a novel Customized Convolutional Neural Network (CCNN)is efficiently proposed. This modifies the elemental form of structurally based design of the convolutional network in such a manner to recognize and classifies the malware images into different family. A Leaky Rectified Linear Unit (Leaky RELU)is incorporated in our architecture, which has the aspect of no zero slopes in negative direction. Therefore, it develops the pictures of productivity of 64x64x50. Along with this Kernel Percolate is introduced, it detect an image pixels with a value of 1 along the diagonals of the image. The experiment is conducted over 9339 samples of the 25 families to classify malware pictures. The outcome of the experiment shows that, contrary to other CNN malware classification models, CCNN has been more than 98.81% accurate. Therefore, the suggested CCNN model reflects in conceptual designs the most comprehensive of malware classification systems.
Keywords: Malware classification, CNN, CCNN, Malware visualization, Deep Learning.